Lower limb muscle matters in patients with hypoxic brain injury following out-of-hospital cardiac arrest
Article information
Abstract
Background
There are conflicting results regarding the association between body mass index and the prognosis of cardiac arrest patients. We investigated the association of the composition and distribution of muscle and fat with neurologic outcomes at hospital discharge in successfully resuscitated out-of-hospital cardiac arrest (OHCA) patients.
Methods
This prospective, single-centre, observational study involved adult OHCA patients, conducted between April 2019 and June 2021. The ratio of total skeletal muscle, upper limb muscle, lower limb muscle, and total fat to body weight was measured using InBody S10, a bioimpedance analyser, after achieving the return of spontaneous circulation. Restricted cubic spline curves with four knots were used to examine the relationship between total skeletal muscle, upper limb muscle, and lower limb muscle relative to total body weight and neurologic outcome at discharge. Multivariable logistic regression analysis was performed to assess an independent association.
Results
A total of 66 patients were enrolled in the study. The proportion of total muscle and lower limb muscle positively correlated with the possibility of having a good neurologic outcome. The proportion of lower limb muscle showed an independent association in the multivariable analysis (adjusted odds ratio, 2.29; 95% confidence interval, 1.06–13.98), and its optimal cut-off value calculated through receiver operating characteristic curve analysis was 23.1%, which can predict a good neurological outcome.
Conclusions
A higher proportion of lower limb muscle to body weight was independently associated with the probability of having a good neurologic outcome in OHCA patients.
INTRODUCTION
Out-of-hospital cardiac arrest (OHCA) is one of the major public health challenges. Despite advances in treatment modalities, hypoxic-ischaemic brain injury occurs in a large proportion of cardiac arrest patients, with the proportion of neurologically intact survivors reported to be <10% in most countries [1]. Cardiac arrest, in which the circulation by the heart is stopped, causes hypoxic-ischaemic damage to multiple vital organs. Among them, hypoxic-ischaemic brain injury is one of the most important factors associated with poor outcome of patients [2,3]. The degree of brain injury in OHCA patients is largely determined by the appropriateness of initial treatment immediately after cardiac arrest, but baseline characteristics of patients, such as age, sex, comorbidities, and body compositions, are also attributing factors [4]. Since assessing the prognosis of OHCA patients at an early stage of treatment is a challenging and important issue, studies have been conducted on the effects of these baseline characteristics on the prognosis of the patients.
Body composition is an index that indirectly reflects patient conditions before cardiac arrests, such as nutritional status or lifestyle, which are difficult to measure objectively. As a representative example, the body mass index (BMI), a simple calculated value using height and weight for estimating the degree of obesity, has been widely studied for the association with the prognosis of cardiac arrest patients [5-9]. Many of these studies have reported that patients with higher BMI are more likely to have better outcomes; however, the results were conflicting. We hypothesized that these conflicting results could be explained by the absence of consideration of detailed muscle and fat composition in BMI. So, we sought to investigate the association of muscle and fat composition with neurologic outcomes at hospital discharge in successfully resuscitated OHCA patients.
MATERIALS AND METHODS
The Institutional Review Board of Seoul National University Bundang Hospital approved this study (No. B-1904-534-302). Written informed consent was obtained from the patient or legal representatives.
Study Design and Setting
The present study was a prospective, single-centre observational study involving OHCA patients, conducted in a tertiary teaching hospital between April 2019 and June 2021. The inclusion criteria were as follows: adult non-traumatic OHCA patients admitted to an intensive care unit (ICU) after achieving a sustained return of spontaneous circulation (ROSC). The exclusion criteria were as follows: (1) patients who did not achieve survival to ICU admission, (2) patients whose baseline cerebral performance category (CPC) was 3 or 4 prior to cardiac arrest, (3) patients with end-stage malignancies, (4) patients whose legal representatives refused to participate in the study, (5) patients who did not have a legal representative to obtain informed consent by the time of ICU admission, and (6) patients whose data acquisition could not be completed because of the required procedure or patient death.
During the study, the emergency medical service (EMS) system was a two-tiered dispatch system in the area including the study institution. When a patient suspected of cardiac arrest was reported, the two nearest available paramedic teams were dispatched to the scene. EMS provided basic life support, and if available, advanced life support (ALS) was dispatched. The ALS included securing an advanced airway and intravenous epinephrine administration under direct medical control through video call. When ROSC is achieved at the scene, the patient was transferred to an emergency department (ED). If the patient was not resuscitated, the patient was transferred to the ED while continuing cardiopulmonary resuscitation (CPR), as per the opinion of the emergency physician [10].
When a patient arrived at the ED without achieving ROSC, ALS was provided in the ED following the American Heart Association guidelines [11,12]. Chest compression was performed using Lund University Cardiopulmonary Assist System 2 (LUCAS-2) device (Physio-Control), an advanced airway was secured, and epinephrine was administered after securing an intravenous line. If the patient achieved ROSC at the ED, hospitalisation to the ICU and post-cardiac arrest care, such as cardiovascular interventions, targeted temperature management (TTM), and hemodynamic support were planned as needed. The TTM was applied with an automated surface cooling device (Arctic Sun) when legal representatives agree to use it, and otherwise, a cold blanket was used as needed. If a patient presented to the ED with ROSC, admission to the ICU and post-cardiac arrest care were initiated forthwith. While ICU admission and post-cardiac arrest care were planned.
Bioelectrical Impedance Analysis
InBody S10 (InBody CO.), a bioimpedance analyser, was used to estimate body composition of the patients, including muscle and fat mass. InBody S10 assesses the total and segmental body muscle and fat composition through direct segmental multi-frequency bioimpedance analysis [13,14]. It estimates body composition by using the difference in conductivity. Since adipose tissue has relatively lesser water content than do other tissues, conductivity decreases as body fat increases. Based on these measurements, the composition ratio of muscle and fat could be calculated.
A tetrapolar eight-point tactile electrode system was used for measurements (Figure 1). Two electrodes were attached to the distal part of both arms and legs, with the patient in the supine position. For the analysis of body composition at each segment, impedance measurements of six different frequencies (1, 5, 50, 250, 500, and 1,000 kHz) and phase angle measurements of three different frequencies (5, 50, and 250 kHz) were performed in each of five segments of the body (both arms, trunk, and both legs).
Measurements were made in the ED or ICU. If the patient was moved to an available ICU immediately after achieving ROSC, the measurement was performed in the ICU. If the patient stayed in the ED while the ICU was being prepared, the measurement was performed at the ED. Total skeletal muscle mass, upper limb muscle mass, lower limb muscle mass, and total fat mass were measured. If the measurement could not be completed owing to necessary procedures required for the patient, or if the patient died before the measurement was completed after admission, the cases were defined as data acquisition failure.
Clinical Data Collection and Preprocessing
Clinical data were collected according to the Utstein registry templates and recorded on a standardised collection form by a research nurse [15]. Baseline characteristics such as age, sex, arrest location, presence of a witness, whether bystander CPR was performed, whether shock was delivered by automated external defibrillator (AED) with bystander CPR before EMS arrival, the first recorded rhythm, and prehospital low flow time (defined as the total amount of time CPR by EMS) were collected. Arrest locations were divided into public and non-public places. Whether TTM was provided with automated surface cooling device after ROSC, and body type information such as height, weight, and waist-hip ratio measured after admission to the ICU were also recorded. BMI was calculated based on the measured height and weight.
The ratio of total skeletal muscle, upper limb muscle, lower limb muscle, and total fat to total body weight was calculated and recorded using the InBody S10 measurements and body weight of the patient, respectively. As an outcome variable, the CPC assessed by the attending physician at the time of hospital discharge was used; a score of 1 or 2 was defined as a good neurologic outcome.
Sample Size Determination Based on Power Analysis
Sample size was calculated based on data of the association between lean body mass (weight of the entire body excluding fat component) and the outcome of OHCA patients [16]. In this study, a lean body mass ≥48.98 kg was associated with a good neurologic outcome after cardiac arrest, and the proportion of patients with a lean body mass ≥48.98 kg in the two groups according to the neurologic outcome was 58.6% and 23.5%, respectively. A total of 66 patients were required to detect a similar difference with an 85% power at a two-sided alpha level of 0.05.
Statistical Analysis
Patients were divided into two groups based on the neurologic outcome at hospital discharge. For clinical data, continuous variables were expressed as median (interquartile range), and categorical variables were expressed as frequency (percentage), respectively. Wilcoxon rank-sum tests were used to compare continuous variables, and chi-square test or Fisher exact test was used to compare categorical variables.
Restricted cubic spline curves with four knots were used to examine the relationship between total skeletal muscle, upper limb muscle, and lower limb muscle relative to total body weight and neurologic outcome at discharge. Unadjusted odds ratios (ORs) with 95% confidence intervals (CIs) for good neurologic outcome of the patients at hospital discharge were calculated according to the change in the proportion of each body composition. Subsequently, multivariable logistic regression analysis was performed to assess whether each body composition measured and calculated had an independent association with neurologic outcome at the time of discharge. Factors considered to be associated with the prognosis of patients such as age, sex, BMI, arrest location, the presence of a witness, whether bystander CPR was performed, whether shock was delivered with bystander CPR by AED before EMS arrival, the first recorded rhythm, and the prehospital low flow time were included in the multivariable analysis. For the body composition that showed an independent association with the neurologic outcome at hospital discharge in multivariable analysis, cut-off values were determined by Youden index through receiver operating characteristic (ROC) curve analysis, and with the cut-off value, multivariable analysis with the same covariable was performed.
All data processing and statistical analyses were performed using the R software package, version 4.1.3 (R Foundation for Statistical Computing), and a two-tailed P-value <0.05 was considered statistically significant.
RESULTS
A total of 363 non-traumatic OHCA adult patients were treated in the ED during the study. Among them, 297 patients were excluded from the study, and 66 were enrolled (Figure 2). Table 1 shows the clinical characteristics of enrolled patients, divided according to the neurologic outcome at hospital discharge. The incidence of a good neurologic outcome at hospital discharge was higher in younger patients and males. There was no significant difference in BMI or waist-hip ratio between groups. Among the body compositions measured, the proportion of total skeletal muscle and lower limb muscle was significantly higher in patients with good neurologic outcome, and the proportion of fat mass and upper limb muscle had no difference between groups.
Figure 3 shows the restricted cubic spline curves with 95% CI of the association between the proportion of each body composition measured by InBody S10 (total skeletal muscle, upper limb muscle, and lower limb muscle) and the odds of having a good neurologic outcome at hospital discharge. The proportion of total muscle and the proportion of lower limb muscle positively correlated with the possibility of having a good neurologic outcome, but the proportion of upper limb muscle did not have any linear correlation. Table 2 shows the unadjusted and adjusted OR for the association of the possibility of having a good neurologic outcome with each body composition. Among the analysed variables, only the proportion of lower limb muscle showed an independent association with the possibility of having a good neurologic outcome at hospital discharge (adjusted OR, 2.29; 95% CI, 1.06–13.98). Detailed results of multivariable logistic regression analysis on the association between the proportion of lower limb muscle and neurologic outcome, and OR and 95% CI for each variable included in the analysis are shown in Supplementary Table 1.
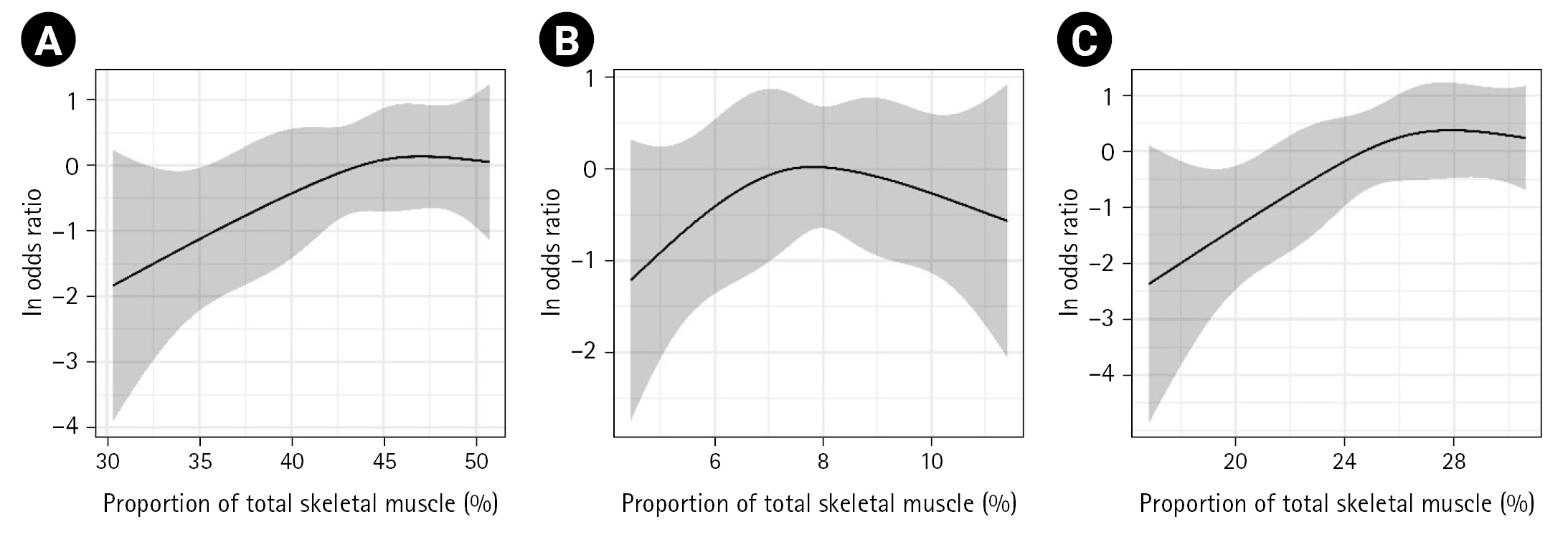
The restricted cubic spline curves for the association between the odds ratio of having good neurologic outcome and (A) the proportion of total skeletal muscle, (B) the proportion of upper limb muscle, and (C) the proportion of lower limb muscle. Each cubic spline curve was drawn with four knots. The dark line indicates the probability of a good neurologic outcome at hospital discharge, and the grey shaded area represents the 95% confidence interval.
The optimal cut-off value of the proportion of the lower limb muscle calculated through ROC curve analysis, which can predict a good neurological outcome, was 23.1%. (Supplementary Figure 1). In multivariable logistic regression analysis, the adjusted OR of the proportion of lower limb muscle ≥23.1% for a good neurologic outcome at hospital discharge was 563.74 (95% CI, 1.86–2.94e8).
DISCUSSION
Results of the present study suggest that a high proportion of lower limb muscle is associated with a good neurologic outcome at hospital discharge in OHCA patients who achieved sustained ROSC. The proportion of lower limb muscle >23.1% showed an independent association with good neurologic outcomes. Considering that this study was conducted with a small population, it was difficult to properly perform subgroup analysis according to patient characteristics. However, because the association between body composition and the prognosis of OHCA patients may vary depending on the clinical characteristics, body composition according to neurologic outcome is additionally assessed in each subgroup. To assess differences according to patient characteristics, body composition was additionally compared in subgroups according to age, sex, BMI, first recorded rhythm, and whether TTM was applied. Subgroups according to age were divided based on the age of 65, and subgroups according to BMI were divided into underweight (BMI <18.5 kg/m2), normal weight (18.5≤ BMI <25 kg/m2), and overweight (25 kg/m2≤ BMI). Supplementary Tables 2-6 show the body composition of patients in each subgroup. In subgroups according to clinical characteristics, differences in lower limb muscles between the two groups according to neurologic outcome were shown in patients with young age, with high BMI, and with an initial shockable rhythm. However, drawing conclusions about independent associations within each subgroup was difficult from these results.
There have been several studies on the relationship between body composition and prognosis in various conditions, such as cardiac arrest, and many of them focused on BMI. These studies have reported that a higher BMI is associated with better outcomes in critically ill patients, including cardiac arrest [5-7,17]. These results, which are contrary to the general negative perception of obesity, coined the expression "obesity paradox." However, these results were not always consistent. According to a meta-analysis that investigated the association between BMI and mortality in critically ill patients, many included studies showed inconsistent results for statistical significance [18]. Furthermore, in the pooled analysis, overweight patients with a BMI of 25 to 29.9 kg/m2 were associated with low mortality, but severely obese patients with a BMI >40 kg/m2 did not.
These inconsistent results also have been reported in studies on cardiac arrest patients. Several studies have suggested that a higher BMI is not consistently associated with a better prognosis [5,8]. Moreover, a recent study reported that a higher BMI might be associated with a poorer prognosis in cardiac arrest patients [9]. In accordance with the inconsistency, our study showed no significant difference in BMI between the two groups classified by the neurologic outcome.
Beyond the application of the conventional BMI, some studies focused on the proportion of muscle in the body. In efforts to examine the idea that body composition, especially the skeletal muscle mass in the body, would influence the outcome of diseases, some researchers have focused on sarcopenia. Sarcopenia, defined as the lack of muscle mass or muscle strength, and occurring mainly in older patients, has been reported to be associated with cardiovascular or respiratory diseases [19,20]. These studies suggested that skeletal muscle mass has an association with underlying conditions of patients, such as exercising, nutritional status, and the presence of metabolic diseases, that are difficult to assess. In addition, a recent study on patients with in-hospital cardiac arrest reported that skeletal muscle depletion prior to cardiac arrest was associated with higher long-term mortality and poor neurologic outcomes [21]. Additionally, sarcopenia is often accompanied by an increase of adipose tissue in older patients, and this sarcopenic obesity is associated with an increase in mortality of patients [22,23]. In the present study, the proportion of total skeletal muscle mass correlated with a good neurologic outcome of OHCA patients, as demonstrated by the restricted cubic spline curve and univariable analysis, although it was not statistically significant in the multivariable analysis.
The underlying mechanism regarding the association between skeletal muscle mass and the prognosis of OHCA patients is unclear. A potential mechanism proposed in preclinical studies is the association with myokines, which are anti-inflammatory hormone-like cytokines secreted by skeletal muscles [24,25]. Since post-cardiac arrest syndrome is a systemic inflammatory condition similar to sepsis [12], sufficient muscle mass and its myokine secreting capacity before cardiac arrest could ensure anti-inflammation and thus may lead to a favourable prognosis of patients. In addition, myokines have been suggested to have a protective effect against metabolic abnormalities in cardiovascular disease [26,27].
Beyond conventional BMI or the composition of the whole body, some researchers have focused on the composition of each section of the body. These studies reported that the risk of cardiovascular disease decreased as the limbs-to-trunk fat mass or fat-free mass ratio increased [28-30]. Although most of these studies focused mainly on the fat mass of each part of the body, rather than on the muscle mass, the results of these studies have suggested that the decreased limb mass may be related to the higher risk of cardiovascular or metabolic diseases. Another study investigated the association of the circumference of limbs with cardiovascular and all-cause mortality in patients [31]. In this study, the circumference of the calf and thigh was suggested to be related to the prognosis of the patients. The circumference of the calf or thigh may have a correlation with the muscle mass of the lower limb, and from this point of view, these results can be comparable to the findings of the present study. Similarly, another recent study reported that the muscle size of the lower limb was associated with the prognosis of patients diagnosed with coronavirus disease 2019 [32]. The lower limb consists of a large percentage of muscle mass in the body and is highly related to performance, which is difficult to measure objectively.
The present study has several limitations. Firstly, this was a single-centre study with a small sample size. Secondly, although we performed multivariable analysis to correct for several possible confounders, the prognosis of OHCA patients is influenced by various complex factors. Accordingly, unmeasured confounders might have influenced the results. Thirdly, the muscle mass for each region used in the analysis was indirectly measured using the InBody S10. Although there have been several reports supporting the measurement of muscle mass through a bioimpedance analyser such as the InBody S10, the indirect measurement method could be biased. Lastly, owing to the characteristic of the measurement method of the InBody S10 device, whereby electric current does not flow through the head, the muscle mass of the head and neck was not completely included in the analysis.
In conclusion, the results of the present study suggested that the higher proportion of lower limb muscle to the body weight was independently associated with the probability of having a good neurologic outcome in OHCA patients.
KEY MASSAGES
▪ There are conflicting results regarding the association between body mass index and the prognosis of cardiac arrest patients.
▪ In this study, the proportion of lower limb muscle >23.1% showed an independent association with good neurologic outcomes, which could be one reason for the conflicting results..
Notes
CONFLICT OF INTEREST
No potential conflict of interest relevant to this article was reported.
FUNDING
This work was supported by the Seoul National University Bundang Hospital Research Fund (No. 02-2020-0034).
AUTHOR CONTRIBUTIONS
Conceptualization: YHJ, KJL, DKL. Data curation: SMP, CWI. Formal analysis: DHJ. Funding acquisition: DKL. Methodology: SMP, KJL. Project administration: DWK, YHJ, DKL. Visualization: DHJ. Writing–original draft: DHJ, SMP . Writing–review & editing: DKL.
Acknowledgements
None.
SUPPLEMENTARY MATERIALS
Supplementary materials can be found via https://doi.org/10.4266/acc.2022.01389.
The result of receiver operating characteristic analysis.
acc-2022-01389-Supplementary-Figure-1.pdfMultivariable logistic regression analysis for good neurologic outcome at hospital discharge
acc-2022-01389-Supplementary-Table-1.pdfBody composition of patients according to the neurologic outcome at discharge in subgroups based on age
acc-2022-01389-Supplementary-Table-2.pdfBody composition of patients according to the neurologic outcome at discharge in subgroups based on sex
acc-2022-01389-Supplementary-Table-3.pdfBody composition of patients according to the neurologic outcome at discharge in subgroups based on BMI
acc-2022-01389-Supplementary-Table-4.pdfBody composition of patients according to the neurologic outcome at discharge in subgroups based on the first recorded rhythm
acc-2022-01389-Supplementary-Table-5.pdfBody composition of patients according to the neurologic outcome at discharge in subgroups based on whether TTM was applied or not
acc-2022-01389-Supplementary-Table-6.pdf